The Secret Mercor Master Plan
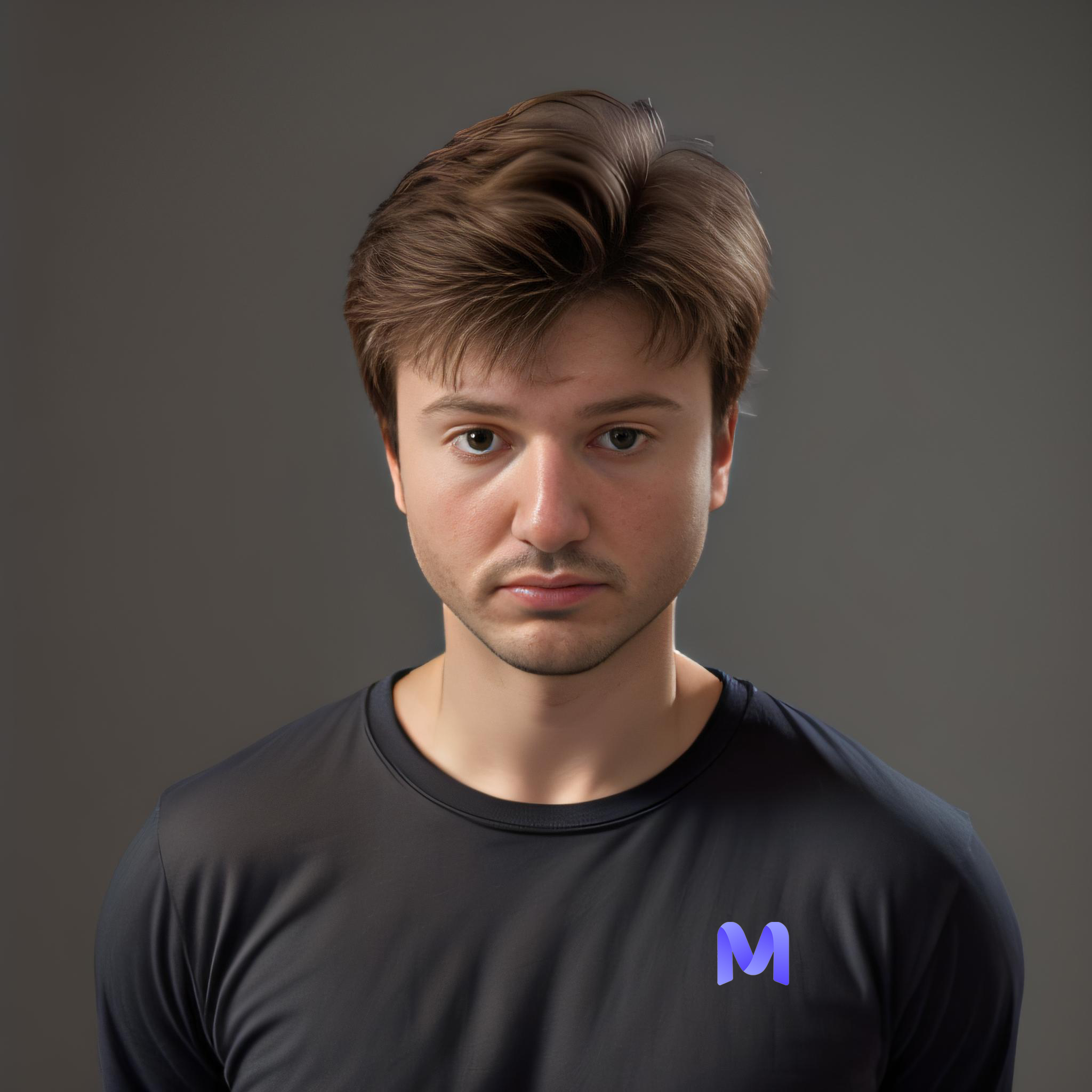
Imagine a world where Jeff Bezos is a hedge fund investor, Howard Shultz is a salesman, and Reed Hastings is a teacher. That was the world we lived in, not so long ago. These are the jobs they were doing before they found the best use for their talents.
We founded Mercor because the labor market is the largest, most inefficient market in the world. Better matching people with the work they do everyday is the largest lever on maximizing global utility. While we gained incredible traction with our initial focus on contracting experts to train AI models, this is only the first step in our plan to solve global labor allocation.
The Wedge
Marketplaces are hard to get off the ground, but if they do take off they become huge. The successful ones have a wedge into a large and pressing unmet need. For Uber, the wedge was black cars. For Airbnb, it was conferences.
We started 2024 in our apartment with no US employees, under $1M in annual revenue, and only seed companies as customers. Last year we grew 6400% and we now work with the most sophisticated technology companies in the world, making us one of the fastest growing companies in Silicon Valley history.
We believe we can create hundreds of thousands of opportunities with AI labs alone, but that pales in comparison to the billions of knowledge work opportunities in the world. The technology that we’re been building is generally applicable.
Structural Inefficiency
Labor inefficiency stems from two structural challenges in the market:
- Fragmentation – Candidates apply to a handful of opportunities and companies consider a fraction of a percent of candidates in the market. This is because matching supply and demand needs to be solved manually (and previously in person). Companies manually review resumes, conduct interviews, and predict who they believe will perform well. Human time is the limiting factor. However, if you can solve this matching problem at the cost of software it allows you to interview everyone, making way for a global, unified labor market that every candidate applies to and every company finds talent from.
- Imperfect Information – When you order a ride on Uber, you know what you’re getting. When you book an Airbnb, the pictures usually do a pretty good job. When you’re hiring someone, it’s extremely difficult to accurately predict how well they will perform. Imperfect human judgement is embedded within every transaction. While LLMs are not perfect at talent assessment, models are quickly surpassing human capabilities. This trend will continue to make transactions more efficient.
Correspondingly, our main objectives are to attract high caliber applicants to come to Mercor and accurately predict candidate’s job performance. Achieving these objectives will solve global labor efficiency more broadly.
Contracting experts to train AI models is the perfect forcing function on these objectives. First, we collect performance data from AI labs within days, compared to the 3 month lag from a traditional enterprise. This allows us to immediately calibrate on the effectiveness of our models and continuously experiment to find the features predictive of success. Second, we need to engage with a broad pool of candidates across all knowledge work roles (law, consulting, medicine, engineering, etc.). This builds the strength of our talent pool across every professional and academic domain. Third, we will service “unreasonable asks” from AI labs like needing to find 300 people in two days. These high volume requests for quality people on extremely short timelines can’t be fulfilled with a services operation. They force us to build the automations at each layer of the engagement process to deliver.
Hiring for All Work
We have the largest comparative advantage from automating talent assessment when the ratio of time spent assessing someone relative to the time spent working with them is the highest. When hiring someone for 5 years, it’s easier to interview them manually. When hiring someone for 5 weeks, efficient matching automation creates a huge comparative advantage. Because of this, we’ve started with shorter duration contract work, but will expand progressively towards longer duration, full-time jobs as our technology matures.
So, in short, the master plan is:
- Hire people to train AI models
- Use those contracts to learn how to predict job performance
- Expand to short-duration contract roles
- Hire people for all jobs
Don’t tell anyone.